Only through clinical evidence – real evidence – can manufacturers prove their medical devices’ safety, performance, and benefit. But when is proof valid enough? In other words, when is there sufficient clinical evidence for authorities and notified bodies to accept?
This article answers the questions and provides a compact introduction to the topic of “clinical evidence.”
1. Relevance of clinical evidence
The requirements of the various stakeholders can only be met with clinical evidence (Tab. 1):
stakeholder group | relevance of clinical evidence |
regulators, notified bodies, authorities (e.g., FDA, regulatory bodies) | The representatives of this stakeholder group demand clinical evidence, for example, when approving medical devices. In this way, they evaluate the safety and benefit of the devices before granting market clearance or registration. |
healthcare providers (hospitals, medical practices, laboratories) | These organizations rely on clinical evidence to evaluate safety and benefits to make informed decisions about medical device choice and use. Evidence-based decision-making ensures patients receive the most appropriate device for their specific medical condition, leading to better treatment outcomes. |
patients | Clinical data, generated mostly through clinical investigations, helps identify and mitigate potential risks associated with medical devices, thereby enhancing patient safety. Clinical evidence helps evaluate the device’s safety profile and provides valuable information to make informed decisions and bring safe devices to the market. |
medical device manufacturers | Manufacturers must provide the clinical evidence required by regulation. It helps them to prove their devices’ conformity and thus create the conditions for product approval. |
2. Clinical evidence: The basics
a) Definitions
Definition according to MDR
The MDR defines the term as follows.
‘clinical evidence’ means clinical data and clinical evaluation results pertaining to a device of a sufficient amount and quality to allow a qualified assessment of whether the device is safe and achieves the intended clinical benefit(s), when used as intended by the manufacturer;
MDR, Article 2 (51)
Definition according to guideline MEDDEV 2.7/1 rev. 4
The guideline does not provide a definition of the term. However, it does define “sufficient clinical evidence.”
an amount and quality of clinical evidence to guarantee the scientific validity of the conclusions
Definition according to FDA
The FDA uses the terms “real-world data” and “real-world evidence.”
Real-world data [RWD] are data relating to patient health status and/or the delivery of health care routinely collected from a variety of sources. Examples of RWD include data derived from electronic health records, medical claims data, data from product or disease registries, and data gathered from other sources (such as digital health technologies) that can inform on health status.
Real-world evidence is the clinical evidence about the usage and potential benefits or risks of a medical product derived from analysis of RWD.
b) Objective of clinical evidence
In (evidence-based) medicine
Die „evidenzbasierte Medizin hat zum Ziel, die ärztlichen Entscheidungsgrundlagen zu verbessern und die Qualität bei Diagnose und Therapie zu erhöhen.“
(Translated: The objective of evidence-based medicine is to improve physician decision-making and enhance the quality of diagnosis and treatment.)
BMG
It aims to achieve this objective by ensuring that medical care is no longer based (solely) on opinions and agreements but on evidence. Evidence is reliable if it is collected using objective scientific methods.
For medical devices
Medical device manufacturers need clinical evidence to demonstrate their medical devices’ safety, performance, and effectiveness (benefits).
Clinical evidence is thus essential for regulators, healthcare professionals, and patients to make informed decisions about medical device selection, adoption, and use. This enables healthcare providers to provide evidence-based care that ensures patient safety and optimizes treatment outcomes.
3. Determine clinical evidence
a) Time and method of determination
Manufacturers are required by law to define the (necessary) clinical evidence for their devices already in the Clinical Evaluation Plan (CEP). This includes defining the scope and quality of the evidence to demonstrate the safety, performance, and risk-benefit balance of the medical device. For example, manufacturers describe the requirements for quality, quantity, completeness, and statistical validity of the data.
According to the MDR, this clinical evidence must be appropriate to the characteristics of the medical device and its intended use and must reflect the state of the art.
Example
The following example shows,
- which data a manufacturer should consider when deciding on clinical evidence,
- why a manufacturer considers these data to be useful and what conclusions a manufacturer expects to draw from them,
- what requirements a manufacturer has for the quality of these data (sources), and
- whether these data were collected with manufacturer’s device or with an equivalent product.
data source | design and justification | own device | equivalent device | not applicable |
non-clinical data | Performance data according to standard Product-specific standards are available, technology has been known for 20 years, and the state of the art is established. The device has no direct clinical benefit/endpoint. | ☐ | ☐ | ☐ |
clinical investigation | Randomized, controlled The state of the art shows data gaps in the performance of the medical device group. | ☐ | ☐ | ☐ |
post-market clinical data – PMS clinical data, complaint and incident reports | Performance data according to standard Product-specific standards are available, technology has been known for 20 years, and the state of the art is established. The device has no direct clinical benefit/endpoint. | ☐ | ☐ | ☐ |
post-market clinical data – Safety database search | see above | ☐ | ☐ | ☐ |
scientific literature | The device has been on the market for 20 years. The risk-benefit ratio is well known. The performance is provided by technical standards; clinical endpoints are only to be confirmed, and the general performance has no gaps. | ☐ | ☐ | ☐ |
PMCF | see above | ☐ | ☐ | ☐ |
b) Challenges in the determination
Challenge 1: Quantifying the evidence
First, manufacturers must determine when they consider clinical evidence to be sufficient and what targets they want to use.
Examples of targets are significance, specificity, and probabilities (such as p-values) that hypotheses are true or not.
Once these target variables are set up, their target values are determined. For example, it must be justified why a p < 0.1 is sufficient.
A distinction is made between levels of evidence in clinical investigations. These are presented below.
Challenge 2: Effort of data collection
The higher the “certainty of evidence” is to be, the more clinical data the manufacturers must collect. Clinical investigations, in particular, are very cost and time-consuming.
The article Clinical data describes which data can be collected and how.
Challenge 3: Coordination with authorities and notified bodies
Notified bodies are required to verify clinical evidence. Therefore, discussions occur regularly, also for existing products.
The notified body shall verify that the clinical evidence and the clinical evaluation are adequate and shall verify the conclusions drawn by the manufacturer on the conformity with the relevant general safety and performance requirements.
Source: MDR, Annex IX, Chapter 2, Part 4.6
Attention: The manufacturer determines the level of evidence.
The MDR states that the manufacturer determines the level of clinical evidence for his device, not the notified bodies!
The manufacturer shall specify and justify the level of clinical evidence necessary to demonstrate conformity with the relevant general safety and performance requirements. That level of clinical evidence shall be appropriate in view of the characteristics of the device and its intended purpose.
MDR, Article 61(1)
Challenge 4: Continuous updating
Rapid technological and medical innovations require continuous evaluation of clinical evidence.
Collaboration between manufacturers, healthcare professionals, researchers, as well as regulators is critical to keep pace with constant innovation. Investing in well-designed clinical investigations, innovative study designs, and using real-world data can improve the quality and efficiency of clinical evidence for medical devices.
4. Evaluate clinical evidence
a) Evaluation of clinical investigations using evidence levels
Clinical investigations are among the most important sources of data. The significance of these studies depends on the level of evidence. A distinction is made between six levels. Level 1 data have the highest significance, and level 6 data have the lowest.
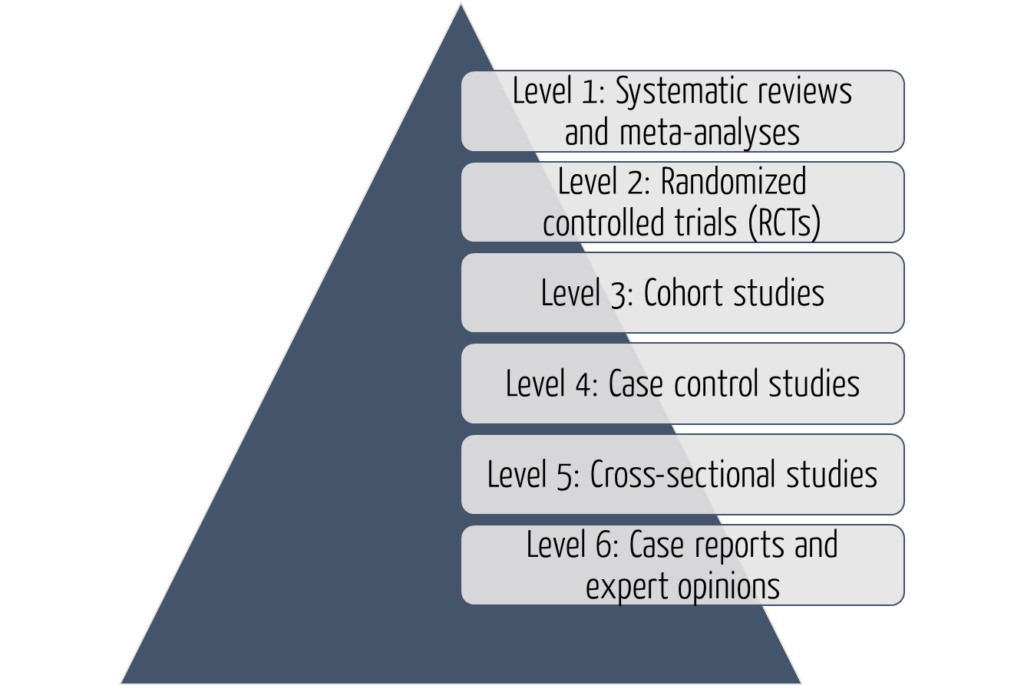
The six levels of evidence help professionals and researchers assess the quality and dependability of evidence presented in clinical investigations. Understanding these levels is essential to making informed decisions in medical practice and evidence-based medicine.
Level 1: Systematic reviews and meta-analyses
At the top of the hierarchy are systematic reviews and meta-analyses, which are considered the highest level of evidence.
Systematic reviews involve a comprehensive identification, assessment, and synthesis of all relevant studies on a given topic.
Meta-analyses go a step further by statistically combining data from multiple studies to supply more precise estimates of treatment effects. This type of study provides a robust summary of the available evidence and is highly valued in evidence-based medicine.
Level 2: Randomized Controlled Trials (RCT)
Randomized Controlled Trials are considered the gold standard for evaluating treatment interventions. In an RCT, participants are randomly assigned to different treatment groups, which allows for the comparison of outputs between groups. RCTs provide convincing evidence of causality and are effective in evaluating the effectiveness and safety of interventions. Well-designed and properly conducted RCTs are generally considered dependable sources of evidence.
Level 3: Cohort studies
Cohort studies are observational studies that follow a group of individuals over an extended time period. They can be prospective (looking ahead) or retrospective (looking back) in design. Cohort studies assess people’s exposure to specific factors or interventions and then follow up on the outputs.
Although they do not involve randomization, cohort studies can provide valuable insights into the natural history of disease, risk factors, and treatment outcomes. However, they are susceptible to bias and confounding factors, which may limit their dependability.
Level 4: Case-control studies
Case-control studies are retrospective observational studies that compare individuals with a particular disease (cases) to individuals without that disease (controls). Researchers evaluate the exposure histories of both groups and determine the association between exposure and disease development.
Case-control studies are useful for studying rare diseases or treatment outcomes, and they are relatively quick and inexpensive. However, they are prone to recall bias and depend on accurate reporting of previous exposures.
Level 5: Cross-sectional studies
Cross-sectional studies are observational studies that collect data from a specific population at a single point in time. Researchers simultaneously collect information on exposure and outcome variables and analyze the associations between these variables. Cross-sectional studies are useful in determining prevalence rates and identifying potential relationships among variables. However, they cannot prove causality or assess temporal associations.
Level 6: Case reports and expert opinion
At the lowest level of the evidence hierarchy, case reports and expert opinions are anecdotal in nature. Case reports describe individual patient cases, while expert opinions are based on the knowledge and experience of recognized authorities in the field. These sources provide valuable insight and can generate hypotheses for further research. Due to the lack of systematic data collection as well as possible bias and subjectivity, they are not considered solid evidence.
b) Clinical guideline evaluation
Differentiation between guidelines and clinical investigations
For clinical evaluations, manufacturers consider clinical investigations as well as clinical guidelines. To this end, they should also evaluate these clinical guidelines for their quality.
Traditional evidence-based medicine addresses a specific clinical question in an individual patient. Guidelines, on the other hand, usually provide decision support for a variety of related clinical situations and patient subgroups.
Important guidelines have been published by Cochrane (only available in German).
Evaluation of guidelines using the S-classification
The manual on systematic search for evidence syntheses and guidelines was produced in its first version as part of the project “Acting on Knowledge” (IIA5-2512MQS006) funded by the German Federal Ministry of Health (BMG) in collaboration between Cochrane Germany and the Institute for Medical Knowledge Management of the AWMF (AMWF-IMWi) and the Medical Center for Quality in Medicine (ÄZQ).
The S-classification helps in the evaluation of guidelines.
level | designation | features |
S3 (high systematics) | evidence- and consensus-based guideline | representative body; systematic research, selection, evaluation of literature; structured consensus building |
S2e | evidence-based guideline | systematic research, selection, evaluation of literature |
S2k | consensus-based guideline | representative body; structured consensus building |
S1 (low systematics) | recommendation for action by expert groups | finding consensus in an informal procedure |
5. Tips to avoid typical mistakes
Tip 1: Recognize and avoid biased results
Clinical investigations play an important role in clinical evidence. Manufacturers should be aware that there are many factors that influence study results, leading to inaccurate, or false conclusions.
These biases can also be related to systematic errors that occur during the planning, conduct, or analysis of a study.
Selection bias
Selection bias occurs when the assignment of study participants to different study arms is influenced by factors related to the output being studied. It can arise when researchers inadvertently favor certain groups or fail to ensure randomization when assigning participants.
This “selection bias” can affect the representation of the target population and lead to biased outputs. To mitigate selection bias, researchers should aim for randomization and ensure that participants are assigned to treatment arms objectively, i.e., without influence or preference, and in a scientifically accountable manner.
Here are other tips to avoid bias:
Information bias
Information bias refers to errors in the measurement or assessment of study variables. It can be divided into two subcategories: Recall bias and measurement bias.
Recall bias
Recall bias occurs when participants in a study selectively remember or report information differently depending on exposure or outcome status.
This bias may be more prevalent in retrospective or case-control studies, where participants may have difficulty accurately recalling past events or exposures.
Measurement bias
Measurement bias occurs when errors or inconsistencies occur in the measurement or assessment of study variables. These can be caused by inaccurate measurement instruments, variability among observers, or misclassification of outputs. Researchers should use rigorous and standardized measurement procedures to minimize measurement errors.
Confounding bias
Confounding bias occurs when an confounding factor, called a confounder, is associated with both the exposure and the output being studied. This association leads to a bias in the true relationship between the exposure and the output, making it difficult to attribute the observed effect solely to the exposure of interest.
The risk of confounding can be controlled by study design (e.g., randomization) or statistical procedures such as stratification or multivariate analysis.
Publication bias
Publication bias is the selective publication of studies based on the direction or statistical significance of their outputs. Studies with positive or significant outputs are more likely to be published than studies with negative or nonsignificant outputs, which may go unpublished or unnoticed.
Publication bias can lead to overestimation of treatment effects and inaccurate representation of the available clinical evidence. To reduce this bias, the publication of all study results should be promoted, despite of their outputs.
Performance bias
Performance bias occurs when the behavior of participants or researchers in a study is influenced by knowledge about the assigned treatment or intervention. This bias can lead to differences in compliance, co-intervention, or assessment of outcomes, which in turn biases study results.
Blinding techniques can be used to minimize performance bias (e.g., double-blind or single-blind designs): Neither participants nor researchers know the treatment assignment.
Reporting bias
Reporting bias refers to selective or incomplete reporting of study results. It can occur when researchers emphasize certain outputs or analyses over others or when they conceal adverse events or secondary outcomes. Reporting bias can affect the overall interpretation of study results and compromise the transparency and reproducibility of research.
Transparent and comprehensive reporting that follows established guidelines, such as CONSORT (Consolidated Standards of Reporting Trials), can help reduce reporting bias.
Cochrane has published a manual on bias assessment (only available in German) that describes the different types of bias and ways to avoid them.
Tip 2: Evaluate literature in a structured way
A structured description of literature sources helps to assess studies in a comprehensible and complete manner. Thus, it is indispensable in determining clinical evidence.
The following points, among others, can be used to guide the structured description:
- Identification of the study
- Description of the study, e.g., objective, level of evidence, number of cases, impact factor, devices used, patient population, etc.
- Assessment of the outputs, e.g., bias, confounders, statement (e.g., about performance)
The Johner Institute uses tools to collect and analyze data in a systematic and categorized way. We are happy to support you in using these as well. This will save you effort and increase the quality of your clinical evaluations.
Tip 3: Determine and ensure the quality of all data
Manufacturers must review not only clinical investigations and the literature for evidence but also data sources such as PMS data, pivotal data, bench tests, and experimental studies.
Define testing criteria
Establishing testing criteria can be used to assess data quality. Typically, manufacturers use a subset of the following criteria to make reliable decisions:
- Completeness
- Uniqueness
- Correctness
- Timeliness
- Accuracy
- Consistency
- Freedom from redundancy
- Relevance
- Uniformity
- Dependability
- Comprehensibility
It is easier to start with a few relevant criteria. As you gain experience and depending on the context, you can add to them piece by piece.
Applying testing criteria
The following diagram shows an example of how the criteria can be applied in practice.
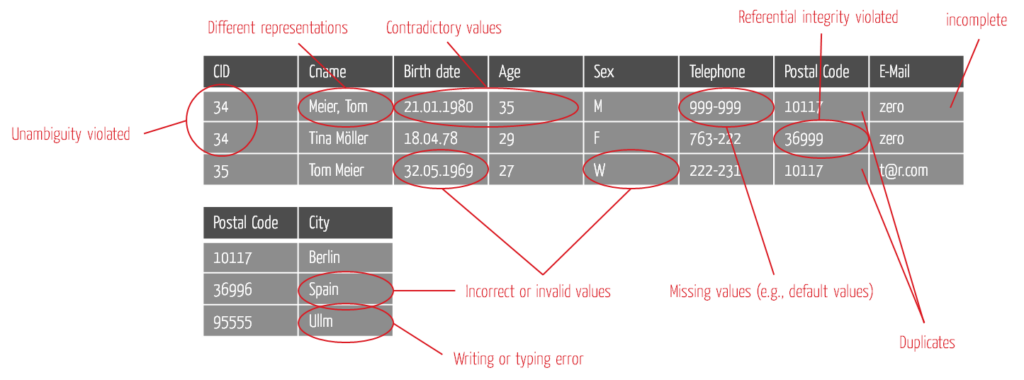
5. Summary and conclusion
Clinical evidence is of major importance in evaluating the safety, performance, and benefits of medical devices. Through preclinical studies, clinical investigations, and observational studies, clinical evidence provides valuable insights for manufacturers, regulators, healthcare professionals, and patients.
By facilitating informed decision-making, enhancing patient safety, and optimizing treatment outcomes, clinical evidence plays a central role in the improvement of medical devices and, thus, the improvement of patient care.
The Johner Institute’s clinical experts can help you to determine clinical strategy and to describe a Clinical Evaluation Plan (CEP). The individual steps include:
- Determine the state of the art
- Derive clinically relevant parameters to derive clinical benefit
- Establish acceptance criteria to achieve the necessary clinical evidence
- Find methods to demonstrate that these acceptance criteria are met
This gives you all the information you need to fulfill your clinical evaluations. You can be confident you have met the regulatory requirements for CEP. Get in touch!